JULO is a financial startup that’s trying to solve the problem of financial inclusion in Indonesia.
“A lot of people in Indonesia don’t have access to traditional financial services,” said Martijn Wieriks, Chief Data Officer at JULO. “Banks have a difficult time underwriting them because they don’t have traditional financial track records. So they end up being excluded.”
JULO is working to change that by using proprietary credit models that leverage alternative data sources like mobile device and health insurance usage.
Credit scoring is at the center of JULO’s business model—making data a key element of its growth and success.
Streamlit has been a key part of JULO’s growth, empowering developers to deliver complex, interactive data visualizations so that stakeholders can quickly make data-backed business decisions.
How JULO went from manual underwriting to automated credit scoring and a 22-member data team
Here’s more about JULO’s unique business model—and how data has been central to its success.
Step 1: Manual underwriting and creation of the first data warehouse
JULO started by building a customer base, a tool kit, and a foundation for their first data warehouse. Agents did the underwriting manually by relying on the data team to collect data and aggregate it into reports.
Step 2: First automated credit model
After JULO collected sufficient data for a year, they built their first credit model iteration. Suddenly they could automate everything.
“Seeing it in practice was amazing,” Martijn said. “We were making the right predictions and enabling the business to make fast credit decisions while carefully managing risk. It was a wow moment.”
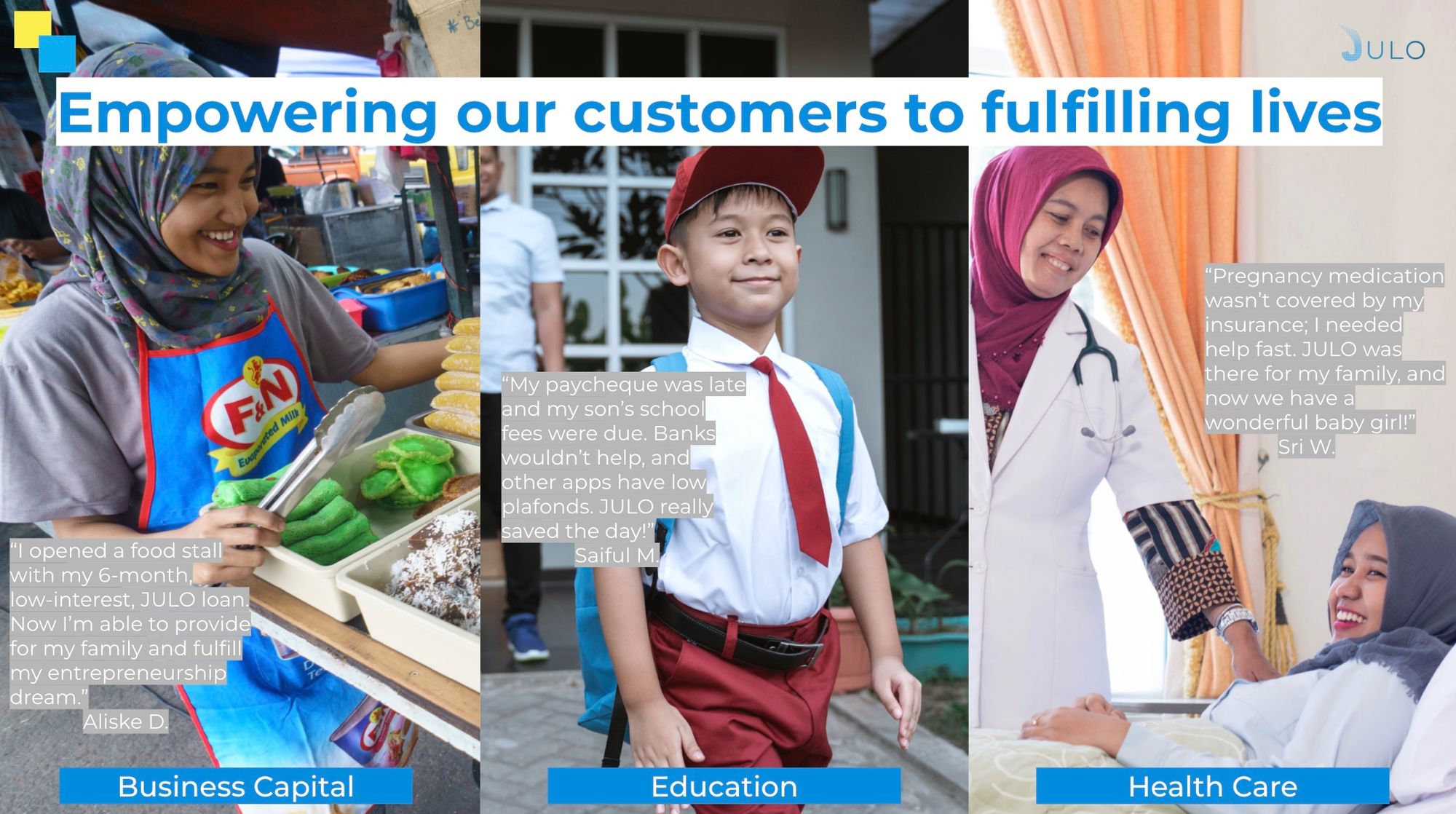
Step 3: Building the development team
“There's a big talent gap in Indonesia,” Martijn said. “Instead of competing with larger companies, we decided to work with talented new grads. We developed a program to train new hires and get them up to speed in 3-6 months.”
JULO is now at 22 people on the data team and is continuing to grow.
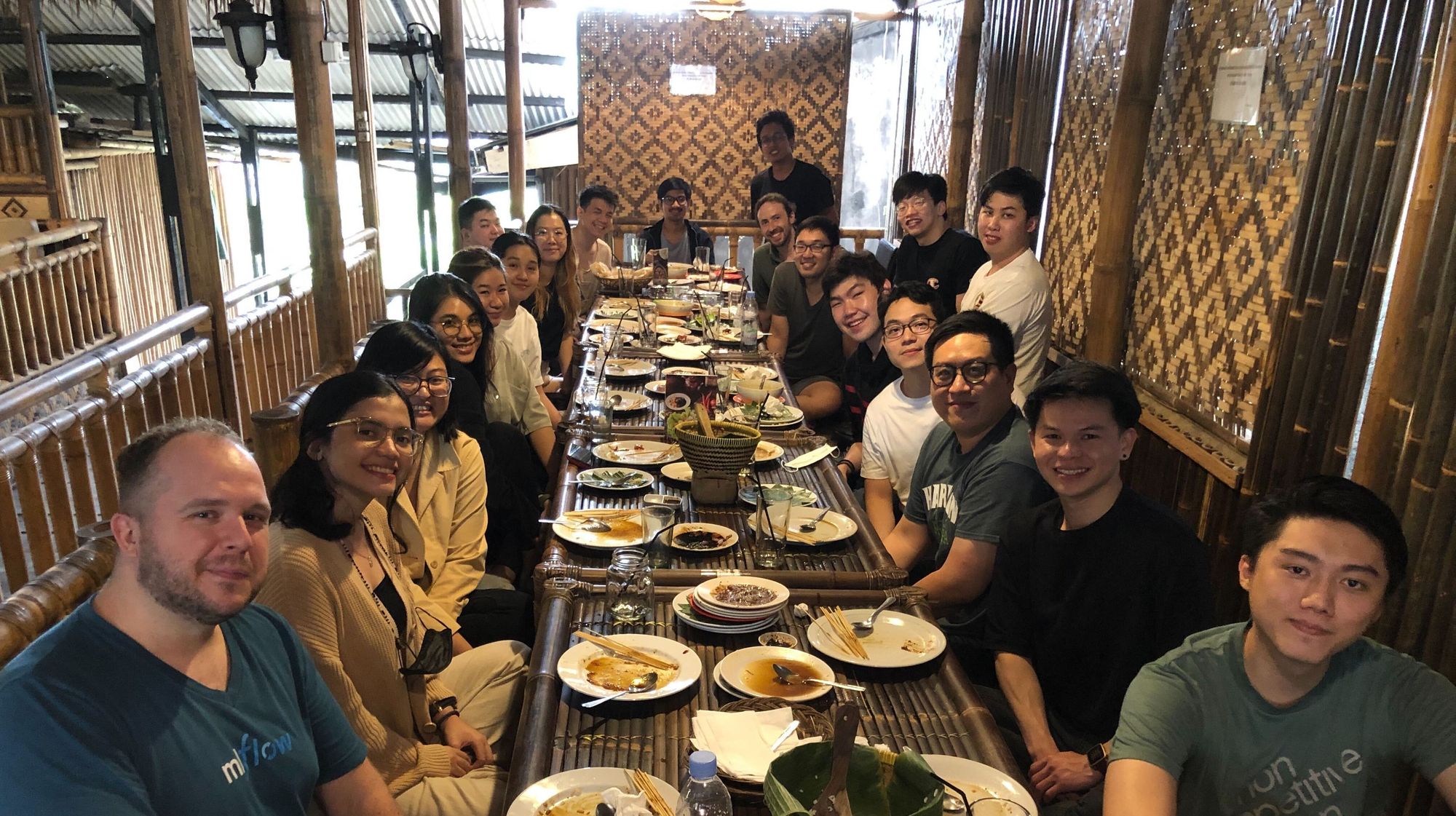
Step 4: Growing the customer base and improving the model over time
Once JULO’s use cases expanded, their technology stack improved and they started getting more customers. This growth posed new challenges.
“The more data variables we added to a machine learning model, the less transparent it became,” Martijn said. “It was important to understand how a machine learning model makes decisions, especially in credit scoring and lending. Because we didn’t want to unfairly bias specific groups.”
Interactive Streamlit dashboards have enabled the finance team to review and plan different strategic scenarios more easily (credit: Darwin Natapradja).
Here is a Streamlit app that simulates credit score performance on different datasets (simulations help data scientists and business users build intuition around metrics):
How JULO discovered Streamlit
“I came across a Medium post and a GitHub repo that mentioned Streamlit,” Martijn said. “It said it was UI for machine learning engineers to create machine learning apps. It didn't click with me then. Six months later I tried it again. There were more app examples. I tinkered with it and was surprised by how quickly I could build a web application. With existing data science skills, like writing Markdown and Python, it was so simple to add interactive components to static code. It was like Jupyter Notebooks on steroids.”
Martijn used Streamlit for his personal projects for two years, then introduced it to his team.
How Streamlit helps JULO grow faster
Data is very important for JULO. It’s their first-class asset, value proposition, and IP. Streamlit helps JULO take care of data, manage bias in models, and demonstrate data science principles to business users and risk managers.
1. Higher velocity
JULO has many active credit lines and needs to report to banks on their financial performance. Martijn’s team used to spend a lot of time on preparing documents, spreadsheets, and slide decks. But they weren’t interactive, so they created a CFO dashboard in Streamlit.
“We could have two conversations of 90 minutes in front of a whiteboard, trying to draw out different risk scenarios to each other. I was there with our CEO, our Business Intelligence Manager, and two data scientists. The whiteboard soon became a complex mess of charts, numbers and variables, which made decision making increasingly difficult. Then over the weekend our BI Manager decided to create this CFO dashboard. On Monday we were able to walk through the same scenarios in an interactive way. It took maybe 10-15 minutes for that to click. We were able to condense 3 hours into 15 minutes to have a breakthrough and get the understanding we needed.”
2. Better decision making
Streamlit lets JULO quickly develop complex and interactive data visualizations.
“We used to build custom Flask apps with Jupyter notebooks and widgets,” Martijn said. “But it's not stakeholder friendly because they don't know how to work with notebooks. Streamlit is a presentation tool. You can serve it as a website. And people can play with it. It's very stakeholder friendly, which is super important because it’s all about putting data solutions into the hands of others. That’s Streamlit’s main value.”
3. Empowered stakeholders
Changing algorithm parameters and doing exhaustive research can help finetune machine learning models. More time spent equals more accuracy.
“Adding more variables may increase the model’s accuracy, but it’ll also make it harder to understand the relationship between them,” Martijn said. “If you only have a customer's age, income, and their latest completed school level, then understanding how a model makes a risk decision is easy. But with a thousand variables, it's a different story. How does each variable affect the score? Is the change positive, negative, or linear? With Streamlit we were able to explore the data, visualize it, and make it accessible.”
What other startups can learn about data from JULO’s story
1. Be patient with lagging data
Any startup that wants to do machine learning needs to collect data for their first model. It takes time. Depending on your use-case your key metrics might be heavily lagging. You’ll be able to see performance data on loans 3-6 months after you've disbursed them. Only then can you say with confidence, “This was a good loan and that was a bad loan.”
2. Visualize data models to get alignment and buy-in
At first we grew slowly. Then suddenly after the first year we had enough data. One rule of thumb in credit scoring is that you need at least a thousand bad loans to build your first model. It took us a while to get there. Once we hit it, we quickly built the first iteration, but we didn’t know if our model would work.
When it did, it was a magic moment. We had a couple of charts and saw people with really high credit scores who had a much lower delinquency rate. That group of people was a lot better than the next, and the next, and the next. We saw a nice sloping line. It was exactly what we were hoping for.
3. Make inclusion a priority
In lending, it has been repeatedly shown that women tend to perform better than men as they're more responsible with money. But if men are over-represented in your data, then a machine learning model may overestimate the risk of women’s delinquency and give them a lower credit score. To detect and manage such unfair bias, we compared groups of women and men so as not to unfairly disadvantage them based on gender.
Wrapping up
“We've been working with Streamlit to solve big problems,” Martijn said. “We've tried other tools, but they haven’t really clicked. Streamlit has become a part of our toolset.”
Thank you for reading JULO’s story! If you have any questions, please leave them in the comments below or contact Martijn on LinkedIn.
Happy Streamlit-ing! 🎈
Comments
Continue the conversation in our forums →